AERA
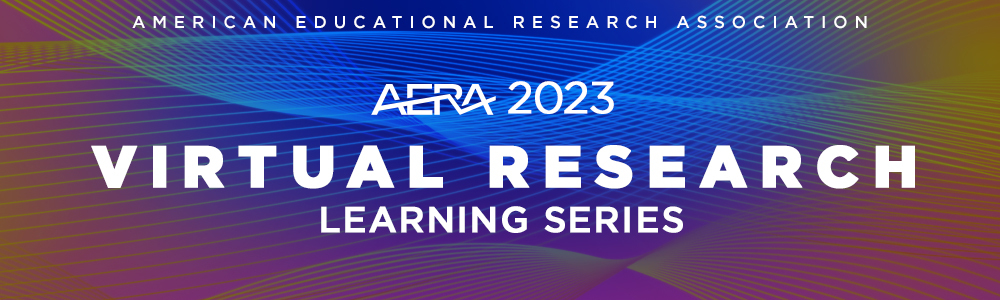
RL2023-3 Text Classification for the Pursuit of Truth with Qualitative Evidence: No-Code Machine Learning Via Latent Code Identification
Recorded On: 07/11/2023
-
Register
- All Users - $55
RL2023-3 Text Classification for the Pursuit of Truth with Qualitative Evidence: No-Code Machine Learning Via Latent Code Identification Tuesday, July 11, 2023 |
INSTRUCTOR: Manuel S. Gonzalez Canche, University of Pennsylvania |
Labeling or classifying textual data is an expensive and consequential challenge for Mixed Methods and Qualitative researchers. The rigor and consistency behind the construction of these labels may ultimately shape research findings and conclusions. A methodological conundrum to address this challenge is the need for human reasoning for classification that leads to deeper and more nuanced understandings, but at the same time manual human classification comes with the well-documented increase in classification inconsistencies and errors, particularly when dealing with vast amounts of texts and teams of coders. This course offers an analytic framework designed to leverage the power of machine learning to classify textual data while also leveraging the importance of human reasoning in this classification process. This framework was designed to mirror as close as possible the line-by-line coding employed in manual code identification, but relying instead on latent Dirichlet allocation, text mining, MCMC, Gibbs sampling and advanced data retrieval and visualization. A set of analytic output provides complete transparency of the classification process and aids to recreate the contextualized meanings embedded in the original texts. Prior to the course participants are encouraged to read these two articles: González Canché, M. S. (2023). Machine Driven Classification of Open-Ended Responses (MDCOR): An analytic framework and free software application to classify longitudinal and cross-sectional text responses in survey and social media research. Expert Systems with Applications, 215. https://doi.org/10.1016/j.eswa.2022.119265 González Canché, M. S. (2023). Latent Code Identification (LACOID): A machine learning-based integrative framework [and open-source software] to classify big textual data, rebuild contextualized/unaltered meanings, and avoid aggregation bias. International Journal of Qualitative Methods, 22. https://doi.org/10.1177/16094069221144940 You can access the articles HERE. |